Google’s journey to a semantic search engine
In this post I would like to discuss what steps and innovations have brought Google closer to the goal of semantic understanding in search since 2010. The article is of interest to SEOs, educators, and journalists who want to learn about search engine technology and evolution of search.
Contents
- 1 What is semantic search?
- 2 Why is semantic search so desirable for Google?
- 3 Google’s steps and innovations towards a semantic search engine
- 4 Infographic “Google’s way to a semantic search engine” for downloading
- 5 Semantic databases or entity index in graph structure
- 6 Vector space analyses and machine learning for better semantic understanding
- 7 Functionality and uses of Natural Language Processing in modern search engines
- 8 Natural Language Processing for building a semantic knowledge base
- 9 Large Language models as an alternative to the classic search index and Knowledge Graph
- 10 More ressources on semantic search
What is semantic search?
A semantic search engine takes into account the context of a search query to better understand the meaning of the search term. In contrast to purely keyword-based search systems, semantic search aims to better interpret the meaning or search intent of the search query and of the meaning of documents. While keyword based search engines work on the basis of keyword-text matching, semantic search engines also consider the relationships between entities for outputting search results.
Why is semantic search so desirable for Google?
There is a common thread running through Google’s major launches regarding search that reveals a clear goal. The complete understanding of all content on the web and unambiguous interpretation of search queries. In order to understand the true meaning of search queries and interpretation of content, Google must
- Clearly identify search queries and their search intent
- Identify entities in search queries and content and organize the index around them
- Identify entities with high expertise, authority and trust (E-A-T)
To achieve these goals, Google needs powerful systems and algorithms that tap into and interpret the complete knowledge of the world of.
Google’s steps and innovations towards a semantic search engine
Google has consistently pursued this goal since the purchase of the semantic database Freebase, the introduction of the Knowledge Graph as a semantic database in 2012, and the launch of the latest ranking algorithm base Hummingbird in 2013. Tapping into the world’s knowledge requires an index built as a graph. While prior to 2012, Google accessed an index similar to a tabular database where information was stored similar to a directory, a graph index can capture and map relationships between information and entities.
Here is a list of the most significant innovations Google has introduced since 2010 on its way to becoming a semantic search engine:
- 2010: Google buys Freebase, a semantic database of structured machine-readable entity data created by Metaweb. The first version of the Knowledge Graph was fed by data from Freebase. In 2014 Freebase was transferred to the Wikidata project. However, of the original 10 million or so records from Freebase, only a portion was transferred. My own record for the entity “olaf kopp”, which I created in Freebase in 2012, was not transferred to Wikidata. I have maintained it manually there. Nevertheless, my data from the former Freebase database is still output in the form of a knowledge panel and extended by further information.
- 2012: Google introduces the Knowledge Graph in the form of Knowledge Panels and Knowledge Cards in search. A knowledge graph is a knowledge database in which information is structured in such a way that knowledge is created from the information. In a Knowledge Graph, entities (nodes) are related to each other via edges, provided with attributes and brought into a thematic context or ontology. More about this below in this article or here >>> Google Knowledge Graph simply explained.
- 2013: Google introduces the Hummingbird update as a new generation of ranking algorithms. The introduction of Hummingbird on Google’s 15th birthday in 2013 was the final launch of semantic search for Google. Google itself has called this algorithm update the most significant since the Caffeine update in 2010. It was said to have affected about 90% of all search queries at launch and was a true algorithm update compared to Caffeine. It is supposed to help to interpret more complex search queries better and to recognize even better the actual sarch intention or question behind a search query as well as to offer matching documents. Also on document level the actual intention behind the content should be better matched with the search query.
- 2014: Google introduces the Knowledge Vault. A system for identifying and extracting tail entities to drive the expansion of the “long tail of knowledge”. Through the Knowledge Vault, Google is able to automate data mining from unstructured sources and could be the foundation for subsequent innovations in Natural Language Processing.
- 2014: Google introduces E-A-T for rating websites in the Quality Rater Guidelines. At first glance, the bridge to semantic search is impossible to draw. Indirectly, however, the entity concept and graph construct of semantic databases provides an ideal basis for a topic-related qualitative evaluation of entities (publishers & authors) and their content in terms of expertise, authority and trust. An entity based index makes it possible to look at entities like authors, publishers, brands, domains … holistically. This is not possible if you only look at single URLs, images … as the classic Google indexes do.
- 2015: Google officially introduces Machine Learning into Google Search with Rankbrain. Via vector space analyses, the search engine wants to better locate search queries and generally terms in a relationship, thematic proximity or context. Among other things, this will allow search queries to be better interpreted in terms of search intent.
- 2018: Google introduces BERT as a new technology for better interpretation of search queries and text. BERT uses Natural Language Processing to better semantically understand search queries, sentences, questions, text segments and content in general.
- 2021: Google introduces MUM as a new technology for better semantic understanding of search queries, questions, content in various forms (text, video, audio, image) and for tapping the “knowledge of the world”. With MUM, Google can add entity information to semanticdatabase(s) like the Knowledge Graph even faster and more extensively. More in my article on search engine land Google MUM update: What can SEOs expect in the future?
- 2023: Under public pressure from the launch of ChatGPT and the introduction of AI-based chatbot functionality at Bing, Google introduces BARD, the PaLM 2 language model, and a beta version of the new Google Search SGE. Google Search is evolving from a search engine to an answer engine with AI-generated answers.
Infographic “Google’s way to a semantic search engine” for downloading
Below is an infographic to explains Google’s way to a semantic search engine for free use for e.g. social media.
Semantic databases or entity index in graph structure
The entities in a semantic database such as the Knowledge Graph are recorded as nodes and the relationships are mapped as edges. The entities can be augmented with labels to, for example, entity type classes, attributes, and information about content related to the entity and digital images such as websites, author profiles, or social network profiles.
Below is a concrete example of entities that are related to each other for clarification. The main entities are Taylor Swift and her boyfriend Joe Alwyn and other entities are the parents, siblings and songs they are semantically related to.
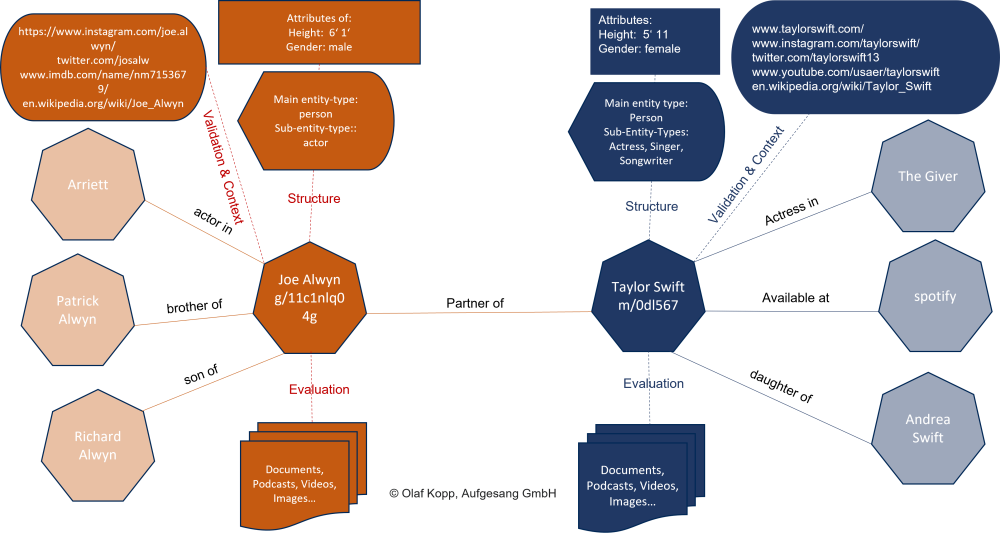
Entities are not just a string of letters but are things with a uniquely identifiable meaning. The search term “jaguar” has several meanings. Thus, the car brand can be meant, the animal or the tank. The mere use of the string in search queries and/or content is not sufficient to understand the context. In combination with other attributes or entities such as rover, coventry, horsepower, car … the unique meaning becomes clear, through the context in which the entity moves. The meaning of entities can be determined by the context in which they are mentioned or used in content and search queries.
Vector space analyses and machine learning for better semantic understanding
Vector space analyses can be used to put search queries and content into a thematic context. One can locate entities, content or search queries in a vector space. The distance between the different terms gives information about the thematic context in which the vectors are used. This way, thematic ontologies or categories can be determined in which keywords or entities are to be located.
The methodology of transforming words into machine-readable vectors (Word2Vec) was officially introduced by Google in 2015 with Rankbrain in order to better understand search queries in particular. Rankbrain was introduced as an innovation for so-called query processing, i.e. the process for interpreting search queries. Rankbrain was also the first time Google confirmed that machine learning is used for Google search.
Functionality and uses of Natural Language Processing in modern search engines
With the launch of BERT in 2018, there was official confirmation from Google that they are using Natural Language Processing for Google Search. Natural Language Processing, as a subfield of Machine Learning, is about better understanding human language in written and spoken form and converting unstructured information into machine-readable structured data. Subtasks of NLP are translation of languages and answering questions. Here it quickly becomes clear how important this technology is for modern search engines like Google.
In general, the functionality of NLP can be roughly broken down into the following process steps:
- Data provision
- Data preparation
- Text analysis
- Text enrichment
The core components of NLP are tokenization, part-of-speech tagging, lemmatization, dependency parsing, parse labeling, named entity recognition, salience scoring, sentiment analysis, categorization, text classification, extraction of content types, and identification of implicit meaning based on structure.
- Tokenization: Tokenization is the process of dividing a sentence into different terms.
- Word type labeling: Word type labeling classifies words by word types such as subject, object, predicate, adjective …
- Word dependencies: Word dependencies creates relationships between words based on grammar rules. This process also maps “jumps” between words.
- Lemmatization: lemmatization determines whether a word has different forms and normalizes variations to the base form, For example, the base form of animals, animal, or of playful, play.
- Parsing Labels: labeling classifies the dependency or the type of relationship between two words that are connected by a dependency.
- Named Entity Analysis and Extraction: This aspect should be familiar to us from the previous papers. It attempts to identify words with a “known” meaning and assign them to classes of entity types. In general, named entities are people, places, and things (nouns). Entities may also contain product names. These are generally the words that trigger a Knowledge Panel. However, words that do not trigger their own Knowledge Panel can also be entities.
Natural Language Processing can be used to identify entities in search queries, sentences and text sections, as well as to decompose the individual components into so-called tokens and set them in relation to each other. Even a grammatical understanding can be developed algorithmically through NLP.
With the introduction of Natural Language Processing, Google is also able to interpret more than just nouns for the interpretation of search queries, texts and language. For example, since BERT, verbs, adverbs, adjectives are also important for determining context. By identifying the relationships between tokens, references can be established and thus personal pronouns can also be interpreted.
Example:
“Olaf Kopp is Head of SEO at Aufgesang. He has been involved in online marketing since 2005.”
In the time before Natural Language Processing, Google could not do anything with the personal pronoun “he” because no reference to the entity “Olaf Kopp” could be established. For indexing and ranking, only the terms Olaf Kopp, Head of SEO, Aufgesang, 2005 and Online Marketing were considered.
Natural Language Processing can be used to identify not only entities in search queries and content, but also the relationship between them.
The grammatical sentence structure as well as references within whole paragraphs and texts are taken into account. Nouns or subject and object in a sentence can be identified as potential entities. Through verbs, relationships between entities can be established. Through adjectives, identify a sentiment (mood) around an entity.

Natural Language Processing can also be used to better answer specific questions, which represents a significant further development for the operation of Voice Search.
Natural Language Processing also plays a central role for the Passage Ranking introduced by Google in 2021.
Google has been using this technology in Google Search since the introduction of BERT in 2018. The Passage Ranking introduced in 2021 is based on Natural Language Processing, as Google can better interpret individual text passages here thanks to the new possibilities.
Natural Language Processing for building a semantic knowledge base
While until now Google was dependent on manually maintained structured and semi-structured information or databases, since BERT it has been possible to extract entities and their relationships from unstructured data sources and store them in a graph index. A quantum leap in data mining.
For this, Google can use the already verified data from (semi-)structured databases like the Knowledge Graph, Wikipedia … as training data to learn to assign unstructured information to existing models or classes and to recognize new patterns. This is where Natural Language Processing in the form of BERT and MUM plays the crucial role.
As early as 2013, Google recognized that building a semantic database such as the Knowledge Graph based solely on structured data is too slow and not scalable because the large mass of so-called long-tail entities are not captured in (semi-)structured databases. To capture these long-tail entities or the complete knowledge of the world, Google introduced the Knowledge Vault in 2013, but it has not been mentioned much since then. The approach to use the complete knowledge available on the Internet for a semantic database via a technology becomes reality through Natural Language Processing. It can be assumed that, in addition to the Knowledge Graph, there will be a kind of intermediate storage in which Google will record and structure or organize the knowledge generated via Natural Language Processing. As soon as a validity threshold is reached, the entities and information are transferred to the Knowledge Graph. This intermediate storage could be the Knowledge Vault.
This also explains why the Knowledge Graph has grown very quickly, especially in recent years.
By mid-2016, Google reported that it held 70 billion facts and answered „roughly one-third“ of the 100 billion monthly searches they handled. By March 2023, this had grown to 800 billion facts on 8 billion entities.”, Quelle: https://en.wikipedia.org/wiki/Google_Knowledge_Graph
More in my article Natural language processing to build a semantic database
Large Language models as an alternative to the classic search index and Knowledge Graph
The rise of large language models (LLMs) can lead a fundamental shift from classical indexes to language models for Google search, as presented in the scientific paper “Rethinking Search: Making Domain Experts out of Dilettantes”. The classical retrieve-and-rank process will be shortened or carried out via language models. Entities, content, text passages … can be stored as embeddings in a vector database. Individual tasks can be handled in fine-tuned language models in addition to a general language model.
This may also have implications for the continued use of the Knowledge Graph as an entity store. Although language models are not knowledge bases, future more powerful language models may approach true semantic understanding and knowledge. Knowledge Graphs could continue to assist in fine-tuning and fact-checking to better understand semantic relationships and mitigate the risk of hallucination.

More ressources on semantic search
- What is semantic search: A deep dive into entity-based search
- Book: Entity orientated search by Krisztian Balog
- Semantic Search by David Amerland
- The dimensions of the Google ranking - 25. April 2024
- Interesting Google patents for search and SEO in 2024 - 3. April 2024
- What is the Google Shopping Graph and how does it work? - 27. February 2024
- “Google doesn’t like AI content!” Myth or truth? - 19. February 2024
- Most interesting Google Patents for semantic search - 12. February 2024
- How does Google search (ranking) may be working today - 4. February 2024
- Success factors for user centricity in companies - 28. January 2024
- Social media has become one of the most important gatekeepers for content - 28. January 2024
- E-E-A-T: Google ressources, patents and scientific papers - 24. January 2024
- Patents and research papers for deep learning & ranking by Marc Najork - 21. January 2024
Ali Jahani
29.06.2023, 11:01 Uhr
You are best bro…